Written by Amy Buckner Chowdhry, Founder of AnswerLab; previously published on AnswerLab's LinkedIn Newsletter on Septem...
Building AI Products That Matter: Why Product Research is Your Secret Weapon
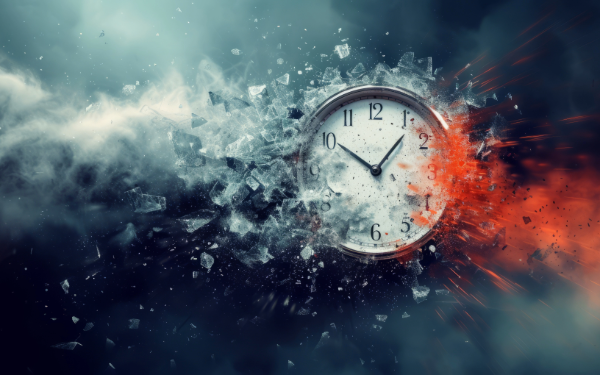
Posted by Ashley Craig on Oct 8, 2024
"No one is using this! What should I do?"
It's a familiar frustration for many product managers. Your AI-powered feature is live, but users aren't engaging with it. The technology is impressive, but it's not solving the right problem.
Why? Because it was built without a clear understanding of what users actually need.
In the rush to stay ahead of AI trends, many teams prioritize engineering over research, leading to products that may be technically advanced but lack real user value. This approach can result in wasted resources and missed opportunities.
The Bottom Line: Product Research Matters for AI Product Success
As a Product Manager, you constantly balance user needs, business goals, and technical feasibility. Incorporating product research into your AI development process isn't just about creating better products—it's about driving real business results:
- Reduced Development Costs: By identifying user needs early and validating concepts before full development, you can avoid costly pivots and rework.
- Increased User Adoption: AI solutions built on user research are more likely to be embraced by your target audience, leading to higher engagement and retention rates.
- Competitive Advantage: Understanding user needs at a deep level allows you to create truly differentiated AI products that stand out in the market.
- Improved ROI: By focusing development efforts on features that users actually want, you can maximize the return on your AI investment.
In short, product research isn't just a nice-to-have—it's a critical tool for ensuring the success of your AI initiatives.
The Numbers Don't Lie: The Impact of Product Research on Product Success
- A study by McKinsey & Company found that companies that prioritize design and user research grow their revenues and shareholder returns at nearly twice the rate of their industry counterparts.
- A report by PwC found that AI could contribute up to $15.7 trillion to the global economy by 2030, but only if it meets real user needs.
- Research by Forrester shows that, on average, every dollar invested in UX returns $100, highlighting the significant ROI of user-centered design.
While not all of these statistics are specific to AI products, they underscore the critical role that user-centered design and research play in the success of digital initiatives. As AI becomes more prevalent in product development, the importance of product research in this field is likely to grow.
The Trap of Building for Trends, Not Users
It's easy to get swept up in AI industry trends—automated assistants, chatbots, predictive analytics—but what happens when those experiences don't connect with your users? Often, they fall flat because they weren't designed with real user problems in mind.
Many products today are designed to mimic what's popular rather than being rooted in solid research about user pain points.
When product teams rely on assumptions or jump on trends, they risk building experiences that are more "cool" than useful. The result is impressive technology that doesn't get adopted.
A prime example of this is Google Glass, launched in 2013. Positioned as a revolutionary wearable technology, Google Glass was meant to bring augmented reality to the masses. It allowed users to access the internet, take photos, and receive notifications through a head-mounted display.
However, despite significant hype and Google's technological prowess, Google Glass failed to gain traction in the consumer market and was discontinued in its original form in 2015. This high-profile failure illustrates several key points:
- Lack of Clear User Need: While technologically impressive, Google Glass didn't solve a clear, pressing problem for most consumers. The product seemed to be more about showcasing technology than addressing user needs.
- Privacy Concerns Overlooked: The ability to record video discreetly led to significant privacy concerns. More comprehensive user research might have flagged this as a major issue earlier in development.
- Social Acceptance Not Considered: The design of Google Glass made users stand out in a way that many found uncomfortable. This social aspect of wearable technology wasn't adequately addressed.
- Usability Issues: Early adopters reported that the interface wasn't intuitive, and the display could cause eye strain, indicating a lack of extensive usability testing.
This case underscores the importance of thorough user research and testing in product development. It's not enough to create technologically advanced products; they must be designed with a deep understanding of user needs, behaviors, and social contexts.
By prioritizing user research and testing over simply following trends, product managers can avoid similar pitfalls and create solutions that are not only innovative but also truly valuable and adoptable by users.
Move Beyond Assumptions with Exploratory Research
So, how do you avoid building AI that misses the mark? The key is research—specifically, exploratory research that goes beyond assumptions and digs into what users truly need. This type of research could include in-depth interviews, contextual inquiries, and diary studies, all designed to uncover user pain points before you start building solutions.
>> Case Study: Exploratory research helps product teams identify the "jobs to be done" that AI can uniquely address. For example, in a recent project, we discovered that users weren't looking for just any automated assistant—they wanted one that could handle complex, nuanced tasks that typical AI tools weren't built to do. By understanding this deeper need early on, the product team was able to develop a more valuable solution.
Validate Concepts with Wizard of Oz Testing
Once you've identified real user needs through exploratory research, the next step is to test your ideas before committing significant resources. This is where Wizard of Oz testing becomes invaluable.
Wizard of Oz testing allows you to simulate AI functionality without building the full system.
By having a human secretly stand in for the AI behind the scenes, product teams can test how users interact with proposed AI features and gather real feedback on conversational flows and expectations.
>> Case Study: In a recent project, we helped a team refine their AI assistant's onboarding flow using Wizard of Oz testing. We simulated user interactions with suggested prompts and clarifying questions. The findings were clear: users appreciated initial guidance but wanted to maintain autonomy. They preferred just enough direction to navigate the system without feeling constrained. This insight allowed the team to design a chatbot experience that felt helpful and intuitive, striking the right balance between assistance and user control to increase product adoption post-onboarding.
Test Throughout the AI Product Lifecycle
Building an AI product isn't a one-time effort—it's a process that demands continuous testing and refinement at every stage of development. Each phase—from early prototypes to post-launch—benefits from different types of user research.
- Early stages: Task-based usability testing ensures users can easily navigate key AI flows.
- Mid-development: Competitive analysis helps refine your features to stand out in the market.
- Post-launch: Engagement testing helps fine-tune the product based on real user experiences.
>> Key Insight: By embedding research at every stage of the product life cycle, you create a product that evolves with your users' needs, improving over time rather than becoming outdated.
Product Research Turns AI into a Problem-Solving Tool
At its core, AI is a tool for solving problems. But without user research, AI risks becoming a solution in search of a problem. Effective research helps ensure that AI is not just functional but deeply aligned with the real needs and behaviors of its users.
Through methods like contextual inquiry, usability testing, and in-depth interviews, researchers can uncover users' pain points, mental models, and expectations, providing the necessary direction to tailor AI functionality to real-world use cases. When user needs are properly identified and met, AI feels intuitive, helpful, and valuable. This alignment ensures that AI solves real problems rather than creating new ones.
>> Case Study: To explore how AI could enhance daily routines, we observed participants struggling with multiple disjointed tools and inefficient processes. The research revealed that users desired better integration and identified key moments for automation to simplify their daily tasks and goal management. These insights directly shaped our client’s product roadmap and design priorities, ensuring that development efforts focused on addressing genuine user pain points and needs.
5 Steps to Integrate Product Research into Your AI Development Process
- Start with user journey mapping: Before diving into AI development, map out your users' current journeys. Identify pain points and areas where AI could add value.
- Conduct stakeholder interviews: Engage with internal stakeholders to understand business goals and technical constraints. This helps align user needs with organizational objectives.
- Implement regular user feedback loops: Set up mechanisms for continuous user feedback, such as in-app surveys or user testing sessions. Use this data to inform ongoing development.
- Create an AI-specific research plan: Develop a research plan tailored to AI development, including methods like Wizard of Oz testing and conversational flow analysis.
- Establish clear success metrics: Define KPIs that measure both user satisfaction and business impact. Regularly review these metrics to guide your AI development strategy.
Overcoming Obstacles: Making Product Research Work in AI Development
As a Product Manager, you might face several challenges when trying to incorporate product research into your development process:
- Time Constraints: "We don't have time for extensive research in our agile sprints."
- Solution: Integrate rapid research methods like guerrilla testing or remote unmoderated studies that can be conducted quickly without disrupting your sprint cadence.
- Budget Limitations: "Product research sounds expensive, and we're on a tight budget."
- Solution: Start small with cost-effective methods like heuristic evaluations or user surveys. As you demonstrate the value of research, you can make a case for larger investments.
- Technical Complexity: "Our AI is too complex for users to give meaningful feedback."
- Solution: Use techniques like Wizard of Oz testing or simplified prototypes to make complex AI concepts accessible to users for feedback
Maximizing Impact: A Framework for Prioritizing Product Research in AI Development
Here's a simple framework to help you prioritize your product research efforts:
Impact vs. Effort: Apply the impact matrix to determine and prioritize your research opportunities.
- High Impact, Low Effort: These are your "quick wins." Prioritize these research activities first.
- High Impact, High Effort: These are your "major projects." Plan these carefully and secure the necessary resources.
- Low Impact, Low Effort: These can be done if time allows, but aren't critical.
- Low Impact, High Effort: Avoid these unless there's a compelling reason.
Critical User Journeys: Focus your research efforts on the most critical user journeys in your AI product. These are typically:
- Onboarding and first-time use
- Core functionality that differentiates your AI product
- Areas with high user frustration or drop-off
Risk Mitigation: Prioritize research on aspects of your AI that, if misunderstood or poorly designed, could lead to:
- Security or privacy concerns
- Ethical issues or bias in AI decision-making
- Significant user errors or misuse of the AI
From Hype to Impact - Research Your Way to AI Success
In the fast-paced world of AI, it's easy to get caught up in the latest trends and buzzwords. But as we've explored, the key to building truly impactful AI solutions lies not in following the hype, but in understanding your users.
By integrating product research throughout your AI development process - from exploratory studies and Wizard of Oz testing to continuous usability assessments - you can:
- Identify the real problems your users need solved
- Validate your AI concepts before committing resources
- Refine your AI solutions based on actual user behavior
- Create AI products that evolve with your users' needs
Remember, AI is not just about impressive technology; it's about solving real problems for real people. By making research your guide, you'll build AI solutions that don't just follow trends but set them - driving user engagement, business success, and true innovation.
***
Ready to transform your AI development process? AnswerLab's research expertise can help you navigate the complexities of user-centered AI design.
Ashley Craig
Ashley is the Head of Product at AnswerLab.related insights
get the insights newsletter
Unlock business growth with insights from our monthly newsletter. Join an exclusive community of UX, CX, and Product leaders who leverage actionable resources to create impactful brand experiences.